Train a Fine-Tuned Neural Network with TensorFlow's Keras API
text
Train a fine-tuned neural network with TensorFlow's Keras API
In this episode, we'll demonstrate how to train the fine-tuned VGG16 model that we built last time to classify images as cats or dogs.
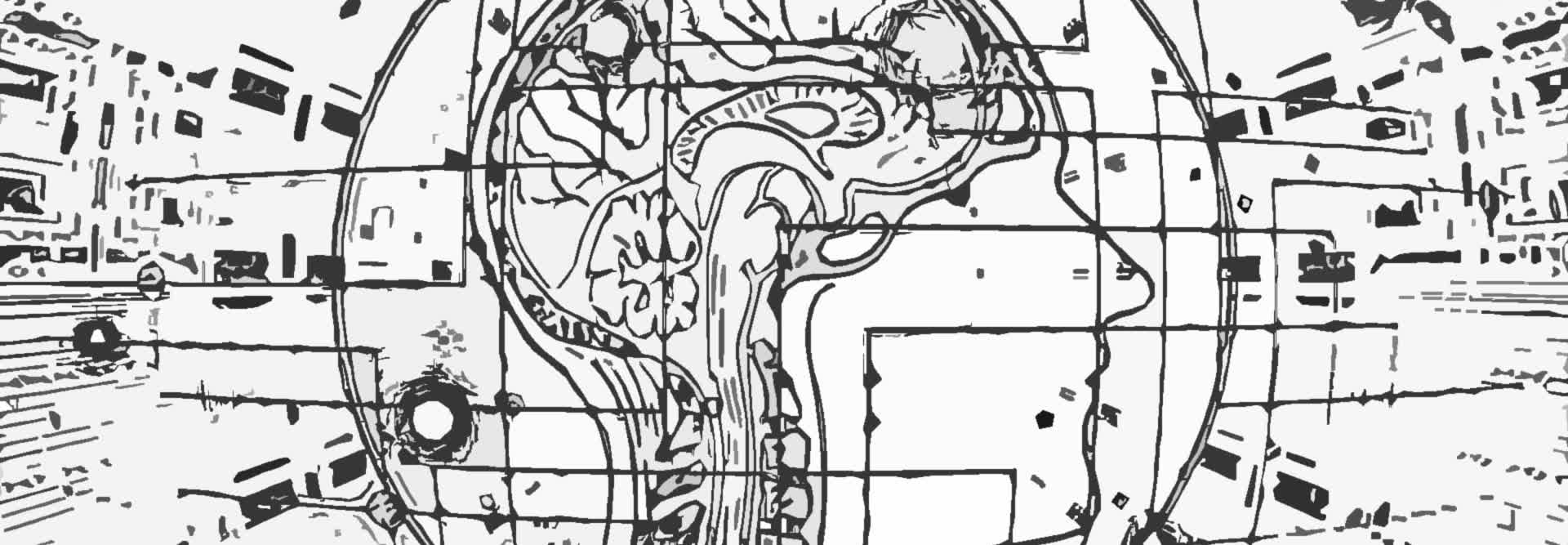
Be sure that you have all the code in place for the model we built in the last episode, as we'll be picking up directly from there.
Additionally, you'll need the code in place from the earlier episode where we organized and processed the image data.
Using our new model, the first thing we'll do is compile
it.
Similar to how we've compiled models in
previous episodes, we'll use the Adam
optimizer with a learning rate of 0.0001
, categorical_crossentropy
as our loss, and
βaccuracy'
as our metric.
model.compile(optimizer=Adam(learning_rate=0.0001), loss='categorical_crossentropy', metrics=['accuracy'])
Now, we'll train the model
using model.fit()
.
Note that the call to fit()
is exactly the same as it was when we used it on the original CNN we built from scratch in a
previous episode, except for we're only running 5
epochs this time, as opposed to 10
.
model.fit(x=train_batches,
steps_per_epoch=len(train_batches),
validation_data=valid_batches,
validation_steps=len(valid_batches),
epochs=5,
verbose=2
)
We pass in our training data and specify all the other parameters in the exact same manner as before. If you need a refresher on these parameters, see the episode where it was covered thoroughly.
Train for 100 steps, validate for 20 steps
Epoch 1/5
100/100 - 18s - loss: 0.2857 - accuracy: 0.8850 - val_loss: 0.1151 - val_accuracy: 0.9500
Epoch 2/5
100/100 - 7s - loss: 0.0754 - accuracy: 0.9800 - val_loss: 0.0826 - val_accuracy: 0.9650
Epoch 3/5
100/100 - 7s - loss: 0.0513 - accuracy: 0.9860 - val_loss: 0.0648 - val_accuracy: 0.9650
Epoch 4/5
100/100 - 7s - loss: 0.0387 - accuracy: 0.9900 - val_loss: 0.0538 - val_accuracy: 0.9800
Epoch 5/5
100/100 - 7s - loss: 0.0272 - accuracy: 0.9950 - val_loss: 0.0487 - val_accuracy: 0.9800
Looking at the results from training, we can see just after 5
epochs, we have some pretty outstanding results, especially when you compare it to the results we got from our original model.
Our accuracy starts off at 88%
and goes over 99%
in just 5
epochs. Similarly, our validation accuracy increases from 95%
to 98%
.
The most noticeable improvement is that this model is generalizing very well to the validation data, unlike the CNN we build from scratch previously.
In the next episode, we'll use this new model to predict on images in our test set and compare the results to the predictions from our original model.
quiz
resources
updates
Committed by on