Loss in a Neural Network explained
text
Loss functions in neural networks
In this post, we'll be discussing what a loss function is and how it's used in an artificial neural network.
Recall that we've already introduced the idea of a loss function in our post on training a neural network. The loss function is what SGD is attempting to minimize by iteratively updating the weights in the network.
At the end of each epoch during the training process, the loss will be calculated using the network's output predictions and the true labels for the respective input.
Suppose our model is classifying images of cats and dogs, and assume that the label for cat is \(0\) and the label for dog is \(1\).
- cat: \(0\)
- dog: \(1\)
Now suppose we pass an image of a cat to the model, and the provided output is \(0.25\). In this case, the difference between the model's prediction and the true label is \(0.25 - 0.00 = 0.25\). This difference is also called the error.
This process is performed for every output. For each epoch, the error is accumulated across all the individual outputs.
Let's look at a loss function that is commonly used in practice called the mean squared error (MSE).
Mean squared error (MSE)
For a single sample, with MSE, we first calculate the difference (the error) between the provided output prediction and the label. We then square this error. For a single input, this is all we do.
If we passed multiple samples to the model at once (a batch of samples), then we would take the mean of the squared errors over all of these samples.
This was just illustrating the math behind how one loss function, MSE, works. There are several different loss functions that we could work with though.
The general idea that we just showed for calculating the error of individual samples will hold true for all of the different types of loss functions. The implementation of what we actually do with each of the errors will be dependent upon the algorithm of the given loss function we're using.
For example, we averaged the squared errors to calculate MSE, but other loss functions will use other algorithms to determine the value of the loss.
If we passed our entire training set to the model at once, then the process we just went over for calculating the loss will occur at the end of each epoch during training.
If we split our training set into batches, and passed batches one at a time to our model, then the loss would be calculated on each batch.
With either method, since the loss depends on the weights, we expect to see the value of the loss change each time the weights are updated. Given that the objective of SGD is to minimize the loss, we want to see our loss decrease as we run more epochs.
Loss functions in code with Keras
Now that we have an idea about what a loss function is, let's see how to specify one in code using Keras.
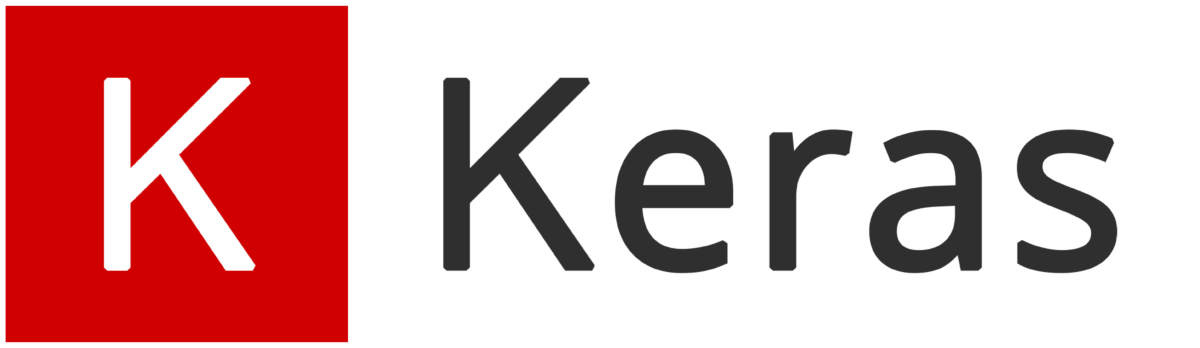
Let's consider the model that we defined in the previous post:
model = Sequential([
Dense(16, input_shape=(1,), activation='relu'),
Dense(32, activation='relu'),
Dense(2, activation='sigmoid')
])
Once we have our model, we can compile it like so:
model.compile(
Adam(learning_rate=.0001),
loss='sparse_categorical_crossentropy',
metrics=['accuracy']
)
Looking at the second parameter of the call to
compile()
, we can see the specified loss function
loss='sparse_categorical_crossentropy'
.
In this example, we're using a loss function called sparse categorical crossentropy, but there are several others that we could choose, like MSE, for instance.
The currently available loss functions for Keras are as follows:
- mean_squared_error
- mean_absolute_error
- mean_absolute_percentage_error
- mean_squared_logarithmic_error
- squared_hinge
- hinge
- categorical_hinge
- logcosh
- categorical_crossentropy
- sparse_categorical_crossentropy
- binary_crossentropy
- kullback_leibler_divergence
- poisson
- cosine_proximity
Hopefully now you have a general idea for what a loss function is, how it works in a neural network, and how to specify one in code with Keras. I'll see ya in the next one!
quiz
resources
updates
Committed by on